Modern Experience. Expert Content.
Effective ethics and compliance training starts with the right foundation. Our SAI360 GRC Platform brings together powerful software and a deep content library to deliver training that’s relevant, engaging and customizable. Tailor courseware to align with your brand, deliver through our LMS or yours, embed disclosures with ease, and track performance with real-time analytics.
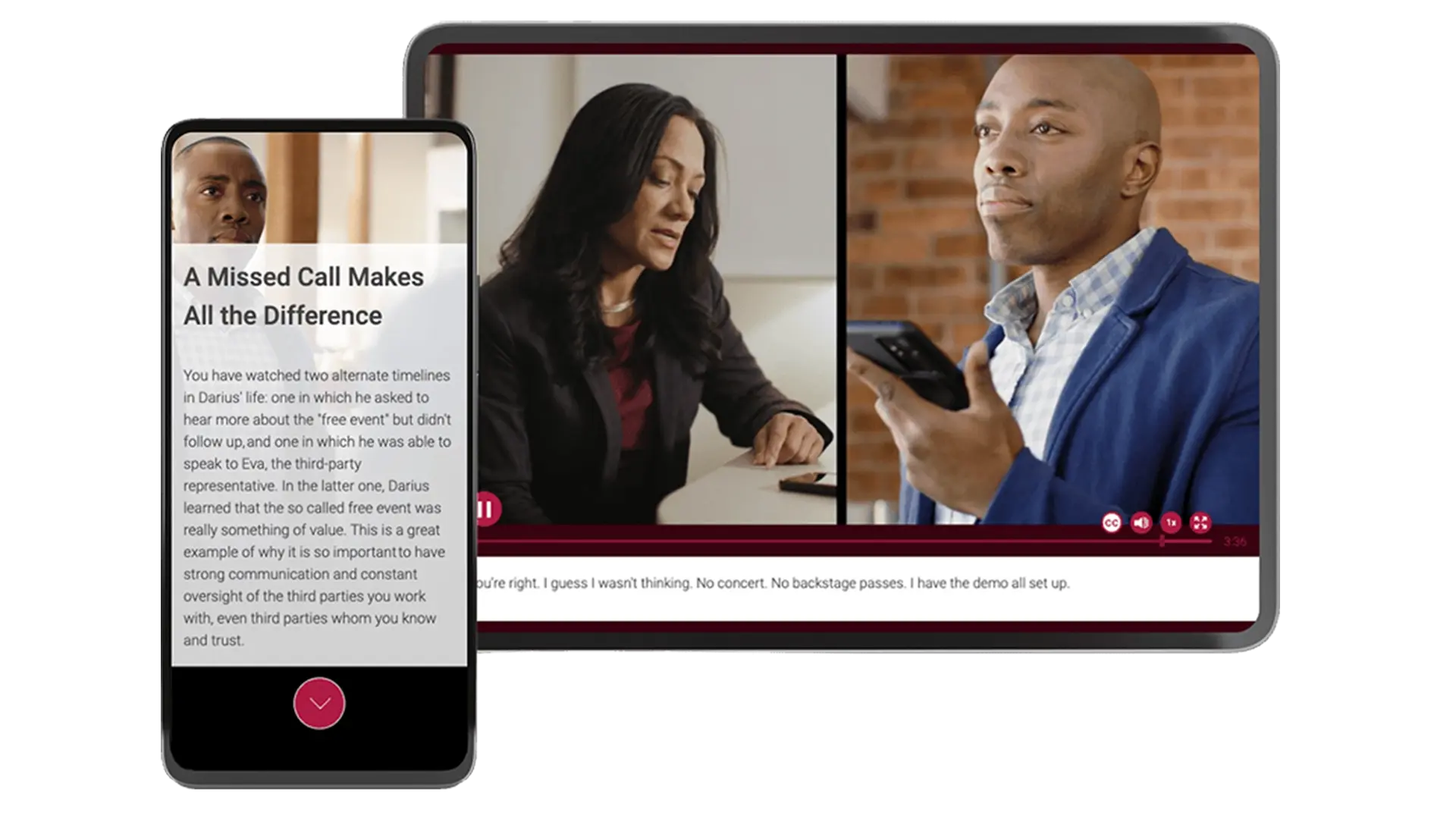
Extensive, Expert-Built Library
Access award-winning ethics and compliance training software that’s trusted, relevant, and ready to deploy across global teams.
- Covers key risk areas, regulatory trends, and code of conduct topics
- Includes microlearning, video, and long-form formats
- Engages over 5 million learners annually across Fortune 1000 companies
Flexible Delivery Options
Train your workforce on your terms—on any platform, anywhere.
- Use our LMS or integrate with yours
- SCORM-compliant, mobile-ready, and built for scale
- Customize content and branding with intuitive editing tools
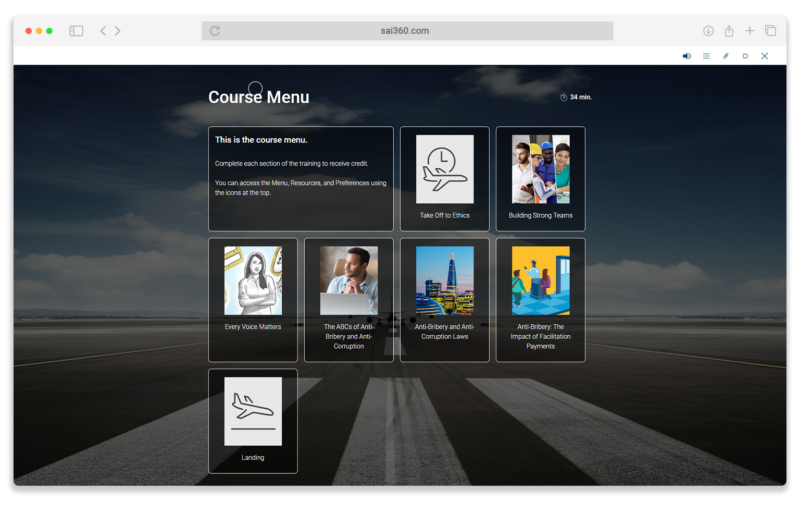
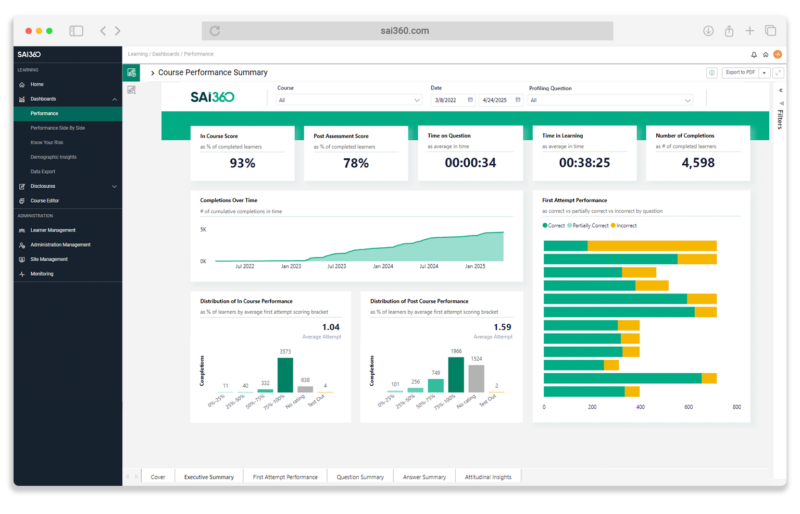
Smart Reporting. Seamless Disclosures.
Gain real-time insight into learners’ ethics and compliance training performance and manage disclosures from one powerful platform.
- Monitor completions, risk signals, and learner trends with real-time dashboards
- Seamlessly collect and manage disclosures like conflicts of interest or gifts
- Generate on-demand reports and support compliance with audit-ready records
Explore The Capabilities
Also on the SAI360 GRC Platform
Deliver a dynamic, branded Code of Conduct that guides behavior across your organization
- 150+ flexible and customizable modules built around your risk areas
- Accessible, interactive, and mobile-friendly
- Connect policies, training, and disclosures within the experience
- Track acknowledgment and reinforce cultural expectations
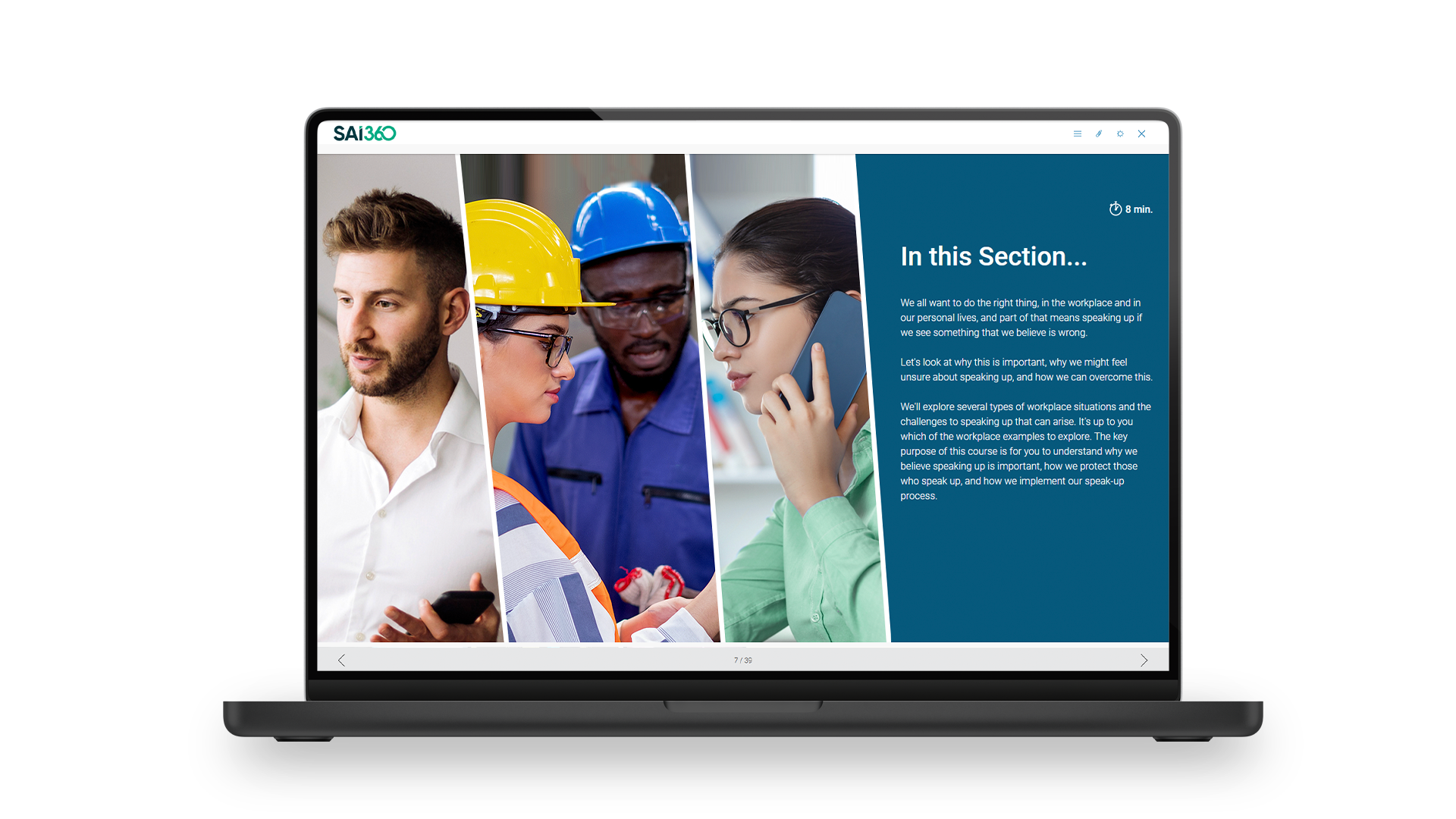
Create, distribute, and manage policies at scale with full visibility and control.
- Draft, approve, and publish policies in one place
- Track attestations and manage version history
- Give employees searchable access to current policies
- Keep policies up to date with automated alerts triggered by changing regulations
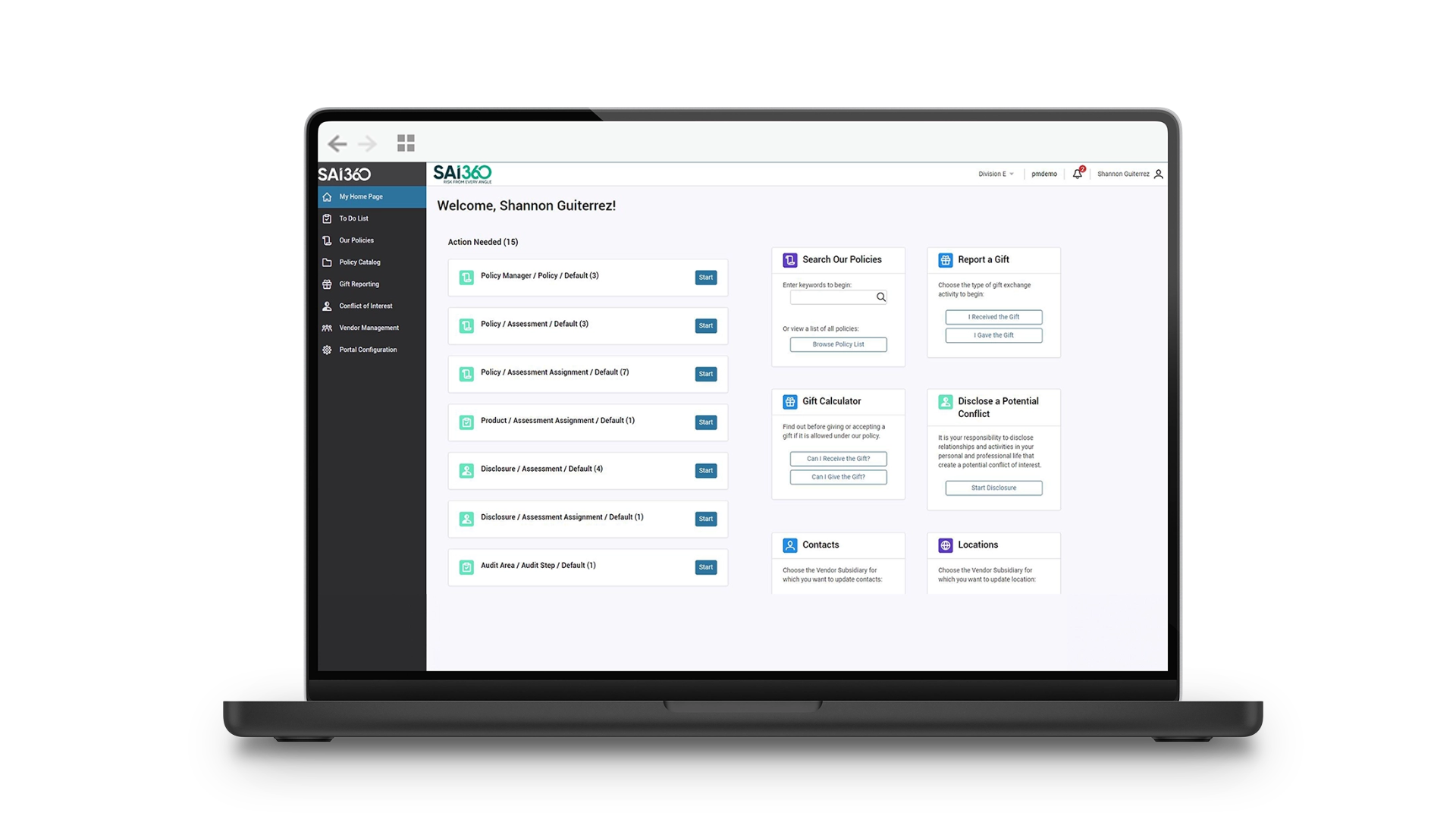
Capture and manage disclosures to surface hidden risks before they escalate.
- Collect disclosures for conflicts, gifts, outside work, and more
- Automate review, escalation, and decision workflows
- Maintain an auditable history of all submissions and actions
- Capture ad-hoc disclosures from intuitive employee portal
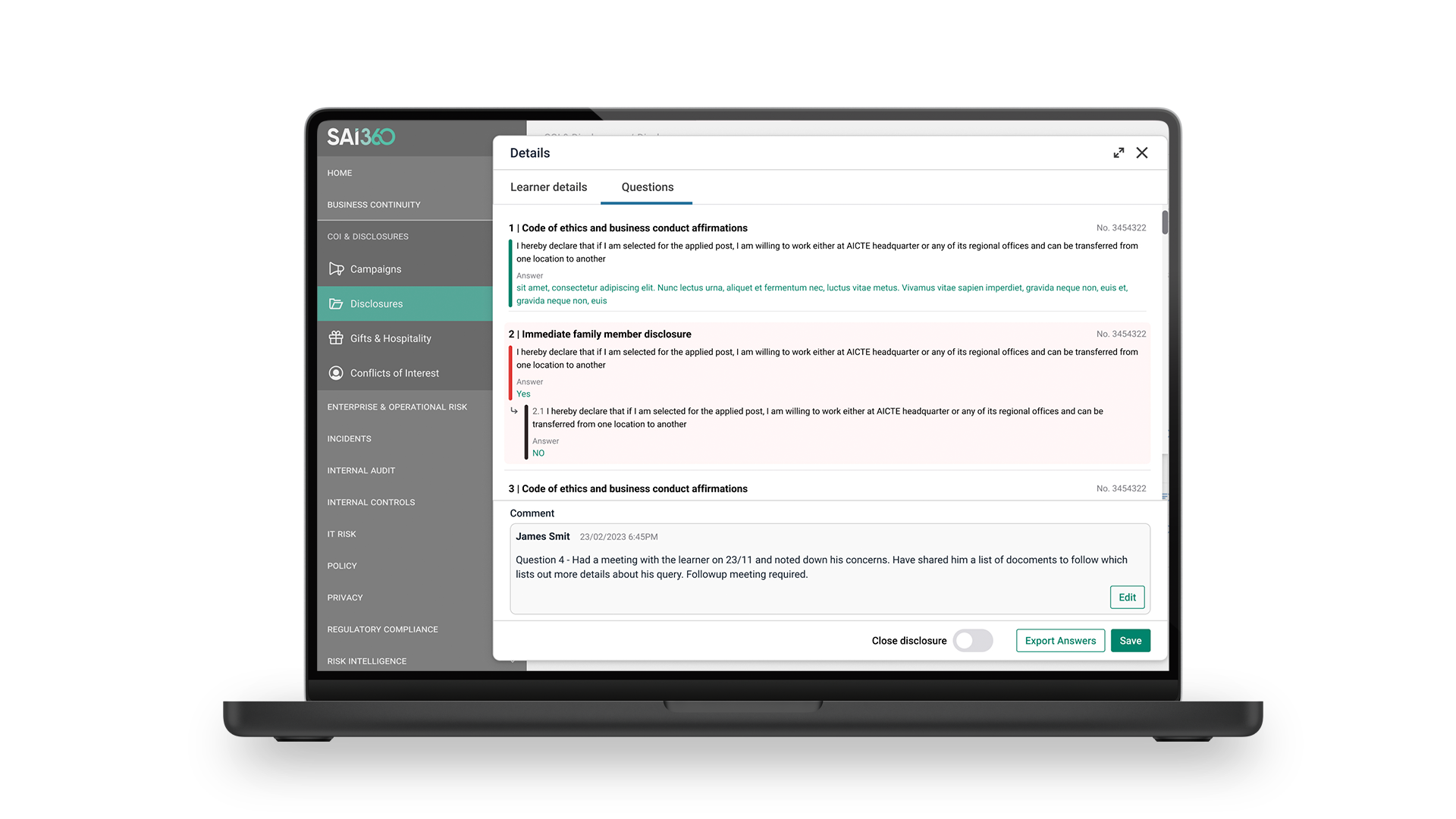
Connect cybersecurity, data, and infrastructure risk to enterprise-level oversight.
- Leverage frameworks like NIST and ISO 27001 to manage IT risk
- Eliminate manual effort with CMDB integration
- Support asset-based risk assessments linked to controls and incidents
- Bridge the gap between IT teams and enterprise risk managers
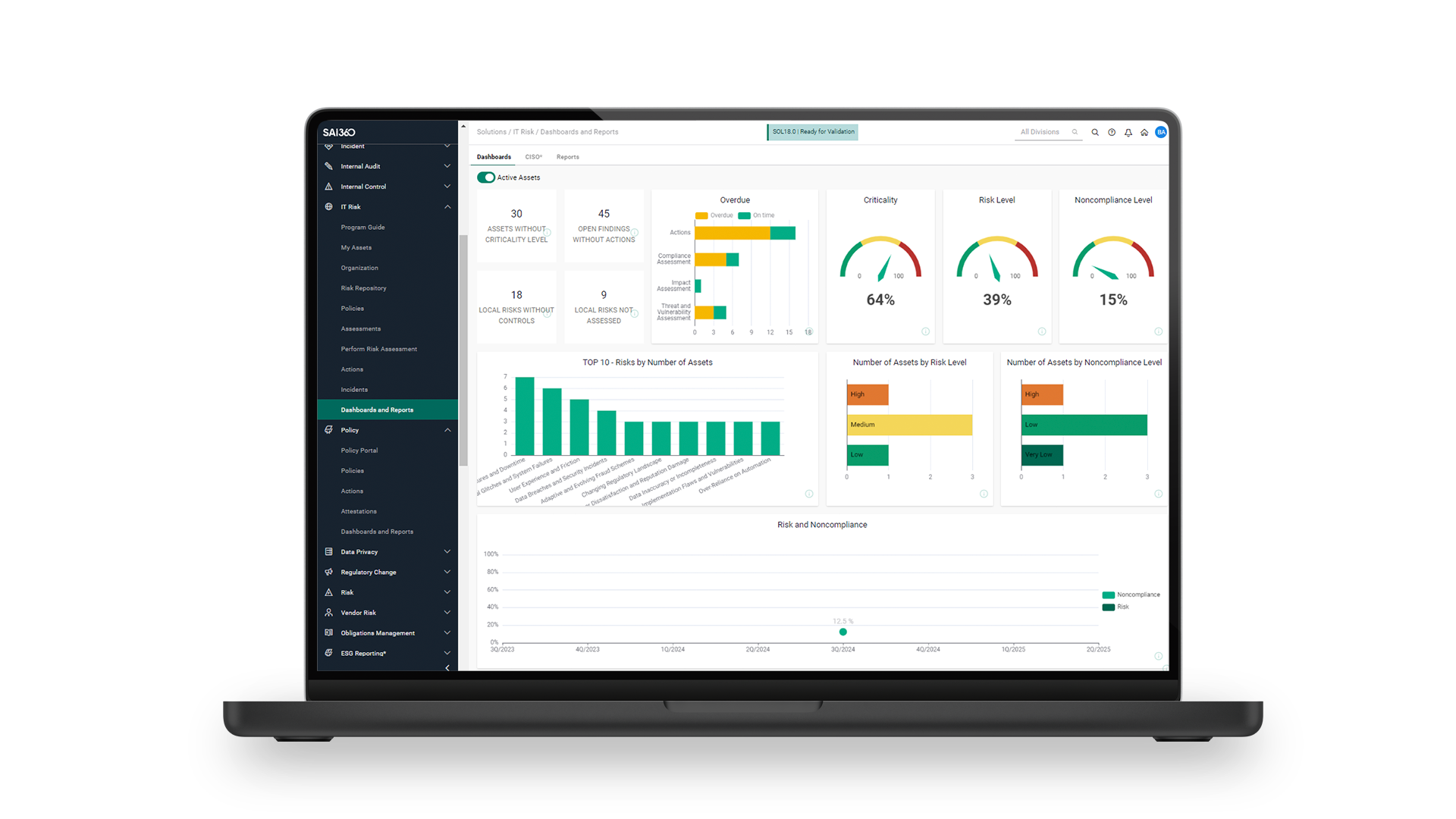
For our integrity learning, we didn’t want to check boxes; we wanted to introduce change behavior and transform culture. We worked with SAI360 to create something new—a portal experience which we created and shared with our employee base within six months.
-Roeland de Wit is the Head of Integrity Operations at ABB